MSE und RMSE - Error estimators in NIR spectroscopy
Both MSE and RMSE are very common error measures, not only in NIR spectroscopy. But how exactly do they work, and in which situations is which of these variants advantageous?
What are error erstimates?
As a rule, NIR spectroscopy is used to search for components in a sample (quantitative analysis) or to determine the sample as a whole (qualitative analysis). For this purpose, models are created in advance, usually by PCR, PLS or with the help of AI.
As a rule, the development of these models always follows the same pattern: first, a collection of suitable samples is compiled and measured in a known, established way. This is done either using already calibrated measuring instruments (e.g. the spectrometer of an external laboratory), or with wet-chemical methods, or by mixing samples yourself so that their composition is clear. Either way, data on the composition of all samples is now available. At the same time, all samples are also analyzed spectrometrically. Once all the data has been collected, this information can be used to calculate which part of the spectral information is particularly relevant for predicting the components of a sample. In the end, a model has been generated that can make statements about the nature of a sample purely on the basis of the spectrum.
Models are just that: models, i.e. they always reduce the complexity of reality. The prediction calculated in this way, regardless of how it was created, will therefore by definition deviate from the real known data.
For example, if we want to compare the established PLS model with our new AI model, we need some kind of metric.
The same applies to the creation of the model itself: Usually, in the course of model calculation, the existing data is split into a training corpus and a test corpus. Only the training data is used to calculate the model. The test data is then used to check whether the model can actually make a good prediction for unknown data sets and has not simply memorized them (this would then be the notorious overfitting: the model is not generally effective, but only for the training data).
In both cases, we want to determine the deviation of the model prediction from the actual values. This is the error measure.
This deviation is of little significance for a single value, as this is where chance plays a maximum role. The data set should therefore be as extensive as possible. The errors per sample are then summarized in different ways.
The two most common variants for this in NIR spectroscopy are the Mean Squared Error (MSE) and the Root Mean Squared Error (RMSE).
Mean Squared Error (MSE)
The MSE is calculated as follows:
MSE = ∑(yi - y^i)² / n
Where
yi
is the actual valuey^i
is the predicted valuen
is the number of samples.
All errors are therefore squared, summed and then subtracted by the number of samples. That means the MSE is the arithmetic mean of the squared errors.
Root Mean Squared Error (RMSE)
The RMSE is calculated in exactly the same way as the MSE, but additionally the square root is taken:
RMSE = √∑(yi- y^i)² / n
MSE vs. RMSE
The effect of squaring in the MSE is that large errors are particularly emphasized in this way, as this means that larger deviations from the actual value are much more significant than smaller deviations. This makes sense insofar as we actually want to have a model whose prediction is as close as possible to reality. However, the same effect can also be a disadvantage if your own data contains outliers that cannot be filtered out in advance. For example, a model could be quite precise, but still have a large MSE error due to a few outliers.
In addition to error weighting, squaring also means that all values always have a positive sign, regardless of the direction of their original deviation.
This also remains the case after the square root of the RMSE; here too, all error measures will be >= 0. However, the special emphasis on large errors no longer exists with the RMSE. Instead, the error measure is now again in the same unit as the calculated variable and is therefore intuitively understandable. For normally distributed data points, i.e. when the data roughly describe a Gaussian curve around the true values, then not only the error magnitude but also the error distribution can be intuitively understood.
Which error measure for NIR spectroscopy?
Whether MSE or RMSE is more recommendable for the evaluation of models in NIR spectroscopy depends somewhat on the location and data quality. In industry, a spectrometric measurement is subject to greater interference than in the laboratory: when measuring above conveyor belts, for example, the distance to the test object - and thus also the radiation intensity of the sample - can vary. Ambient conditions such as temperature or ambient light are less controllable than in the laboratory. And, of course, the material surfaces cannot be prepared during the process and may lead to strong scattering effects if they are in an unfavorable position.
Due to these and other factors, data collected in this way can therefore often contain outliers, although these are also typical of the subsequent application scenario. To ensure that the model to be created is as robust as possible in the field, it is better to optimize with RMSE rather than MSE.
However, if the primary goal is a model that is as precise as possible with the smallest possible error, then MSE is the error measure of choice instead.
You might also like
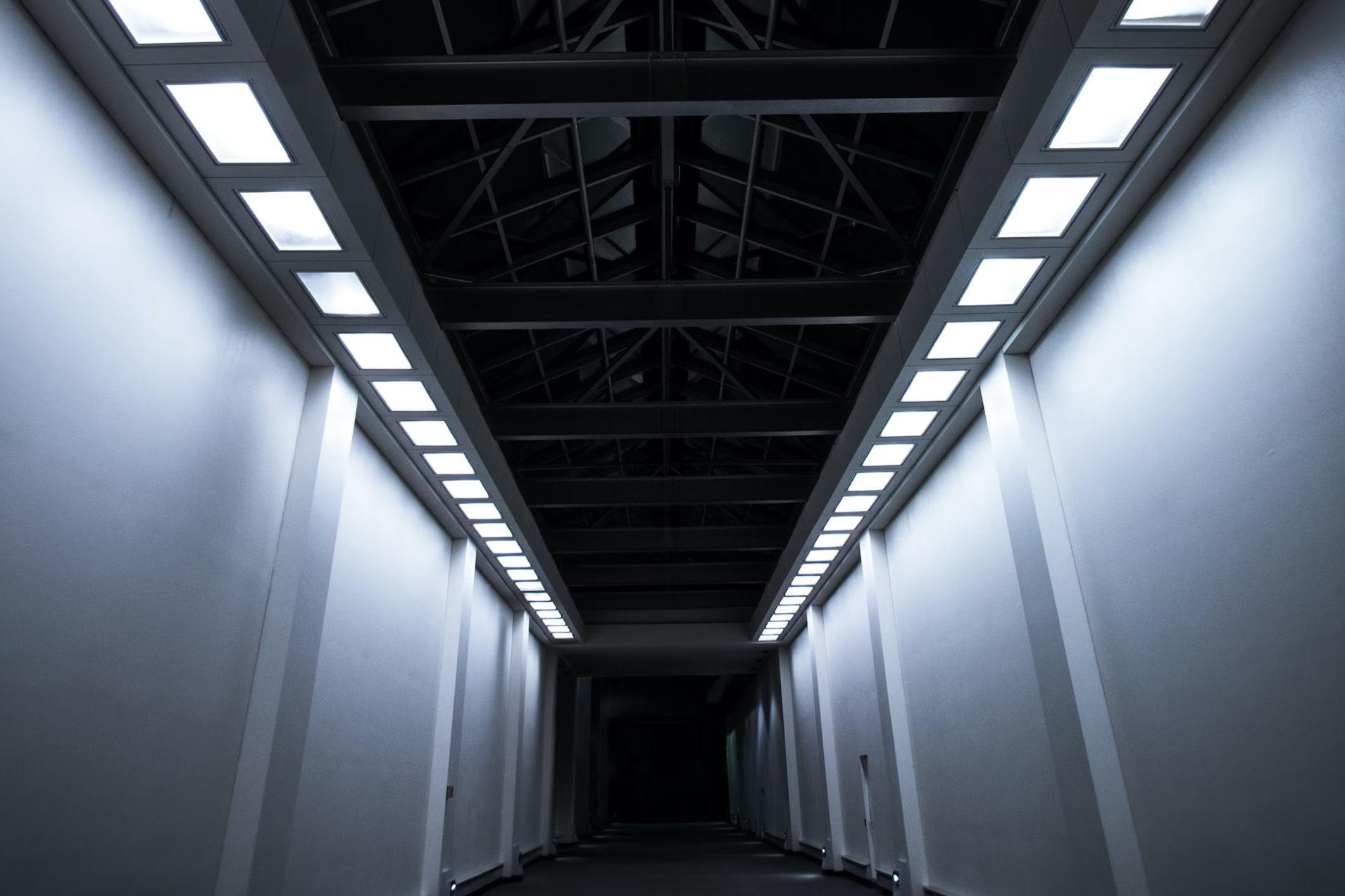
Nits, Lux, Lumen, Candela - calculating with light and lighting
Luminous flux, luminous intensity, luminance and co. - when is which quantity used and how can they be compared?